In the modern business landscape,
data has become the lifeblood of organizations, driving informed decision-making and strategic planning.
As companies increasingly rely on data-driven insights to guide their operations, the quality of the data at hand becomes paramount.
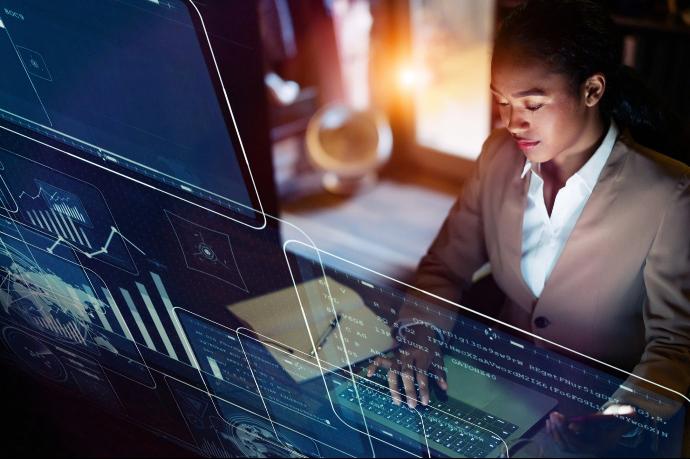
According to 2017 Gartner’s Data Quality Market Survey, bad data costs businesses an average of $15 million annually. The same year, Uber became a casualty losing “tens of millions” of dollars in the end. This arose because of the overcalculation of commission cuts in its accounting software which made the drivers to be underpaid. The error started from an update in 2014 to Uber’s terms of service. As a result, Uber skipped subtracting taxes and fees before collecting a 25% commission. This error made Uber repay its drivers. In the end, it cost Uber $900 per driver running into tens of millions of dollars. This exposes a vulnerable process Uber has in place to check for data quality in its accounting organisatiion; no one at Uber noticed the data error. If checking data had been routine and systematic, the error could have been avoided in the first place. If good data quality assurance practices were effectively in place, this would have triggered an alert that would notify Uber of the incorrect formula for calculating its commission.
Understanding Data Quality
Data quality refers to the accuracy, completeness, consistency, timeliness, validity, uniqueness, and relevance of data and it is critical to all data governance initiatives within an organisation. It is the foundation upon which informed decisions are built. High-quality data ensures that decisions are based on accurate information, minimising the potential for errors and misguided strategies. Inaccurate or incomplete data can lead to faulty assumptions, bad boardroom decisions, and poor outcomes.
The Impact of Data Quality on Corporate Decision-Making
Enhanced Decision Accuracy
In the fast-paced business era, accurate data significantly improves the precision of decision-making, and lack or inadequate of it impedes decision-making. Consider a retail company deciding on inventory levels based on sales data. If the sales data is accurate, the company can optimise its inventory, preventing overstocking or understocking scenarios. Also, deals are struck based on the available data about the business. Many venture capitalists lost their investment when car-hailing companies were making in-root into Nigeria’s Economy because Nigeria’s Internet penetration was very low and the signal strength was not what we have today due to the presence of 4G and 5G. Data available to many of them were the population size and the opportunity to expand and perhaps not considering internet penetration and quality of service by service providers which led to failure of some starter companies in the business.
Mitigation of Decision Biases
Biased or incomplete data can lead to biased decisions. For instance, if a company's data collection disproportionately represents one customer demographic, it may overlook valuable insights from other segments. Data quality efforts help identify and rectify these biases, leading to more equitable decisions. Nokia could not stay withered when Apple entered the industry with consumer preference-the iPhone, which revolutionised the smartphone industry for good because of rapidly changing consumer preferences. Captured consumer preferences are vital to forecasting and making informed long-term business investment and decisions.
Improved Forecasting and Planning
Data accuracy plays a pivotal role in forecasting future trends and planning strategies. Financial institutions rely on accurate historical data for predicting market movements while manufacturing companies use reliable data to anticipate demand fluctuations and adjust production accordingly. Forecasting insights also help companies to formulate plans, improve customer satisfaction, budget for capital expenditures in anticipation of expansion estimate the financial needs of a company and increase the chances of business success.
Challenges in Ensuring Data Quality
Data Collection Challenges
Data collection errors and inconsistencies can arise from manual data entry. Ensuring proper training, validation checks, and error correction mechanisms are essential to maintain data accuracy at the source.
Data Integration and Transformation
Incorporating data from disparate sources can lead to integration challenges. Without standardized transformation processes, inconsistencies may arise, affecting data quality. Robust integration strategies and tools are crucial to ensure coherent data sets.
Data Cleaning and Maintenance
Data quality is an ongoing effort. Over time, data may become outdated or irrelevant. Regular data cleaning and maintenance routines are necessary to weed out obsolete information and maintain the accuracy of the dataset.
Data Quality Assurance Strategies
Establishing Data Governance Policies
Data governance plays a pivotal role in maintaining data quality. Establishing clear data ownership, validation protocols, and maintenance responsibilities within an organization's governance framework ensures data remains accurate and relevant.
Implementing Quality Control Measures
Incorporating quality control checks at different data lifecycle stages helps identify and rectify errors early on. Implementing automated validation processes and conducting periodic data audits contribute to better data quality. This is what Uber should have done to prevent a severe loss.
Data Quality Metrics and Monitoring
Defining data quality metrics, such as accuracy rates and completeness percentages, provides a quantifiable way to measure data quality. Implementing monitoring systems that trigger alerts when data quality thresholds are breached helps ensure ongoing improvement. In 2018, a Samsung Securities employee in South Korea made an error mistaking won (South Korea’s currency) for shares, paying out 1,000 Samsung Securities shares to workers instead of 1,000 won per share in dividends. That single human error cost the technology company $300 million in the end. It was fixed within 37 minutes, but for a short period of time, the company had issued a mind-blowing $105 billion worth of shares. This type of error is avoidable if there are monitoring systems that trigger alerts when thresholds are breached.
Future Trends in Data Quality and Decision-Making
Artificial Intelligence and Machine Learning are poised to play a larger role in enhancing data quality. Automated data validation algorithms can identify discrepancies more efficiently, reducing manual intervention. As data quality improves, predictive analytics gains accuracy. Organisations can use these insights for anticipatory decision-making, aligning strategies with future trends rather than reacting to past data. Making corporations now leverage these for well-researched, evidence-based, and model-proved decision-making.
In the realm of corporate decision-making, the value of high-quality data cannot be overstated. Accurate, reliable, and well-managed data empowers boardroom members to make informed choices, mitigate biases, and enhance planning. Addressing challenges through data governance, quality control measures, and ongoing monitoring ensures that data remains a strategic asset, ultimately contributing to the success and longevity of the business.